Wait – Performance problems writing to an AWS RDS Postgres database?
Haven’t we been here before?
Yes, we had, and I wrote quite a few posts of the trials and tribulations that we went through.
But this is a new problem that we came across that resulted in several messages backwards and forwards between us and Qlik before we worked out the problem.
Core System Migration.
Our organisation has several core systems; making maintenance of them expensive and holding us back in using the data in these systems in modern day tools like advance AI. Over the past several years – various projects are running to consolidate the systems together.
This is all fun and games for the downstream consumers – as they have lots of migration data coming down the pipelines. For instance, shell accounts getting created on the main system from the sacrificial system.
One downstream system wanted to exclude migration data from their downstream data and branch the data into another database so they can manipulate the migrated data to fit it into their pipeline.
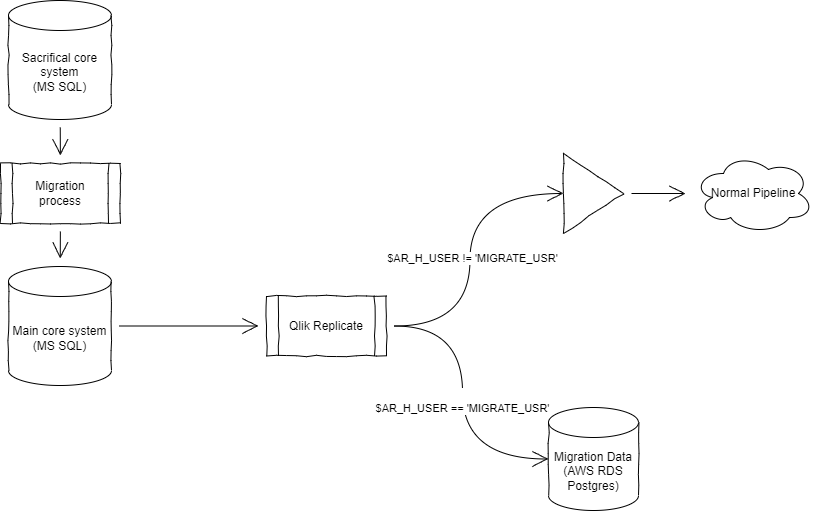
I created the Qlik Replicate task to capture the migration data. It was a simple task to create. Unusually, the downstream users created their own tables that they want me top pipe the data into. In the past, we let Qlik Replicate create the table in the lower environment, copy the schema and use that schema going forwards.
Ready to go we fired up the task in testing to capture the test run through of the migration.
Slow. So Slow.
The test migration ran on the main core system, and we were ready to capture the changes under the user account running the migration process.
It was running slow. So slow.
We knew data was getting loaded as we were periodically running a SELECT COUNT(*) on the destination table. But we were running at less than 20tps.
Things we checked:
- The source and target databases were not under duress.
- The QR server (although a busy server) CPU and Memory wasn’t maxed out.
- There were no critical errors in the error log.
- Records were not getting written to the attrep_apply_exceptions table.
- There were no triggers built off the landing table that might be slowing down the process
- We knew from previous testing that we could get a higher tps.
I bumped up the logging on “Target Apply” to see if we can capture more details on the problem.
One by One.
After searching the log files, we came across an interesting message:
00007508: 2025-02-20T08:33:24:595067 [TARGET_APPLY ]I: Bulk apply operation failed. Trying to execute bulk statements in 'one-by-one' mode (bulk_apply.c:2430)
00007508: 2025-02-20T08:33:24:814779 [TARGET_APPLY ]I: Applying INSERTS one-by-one for table 'dbo'.'DESTINATION_TABLE' (4) (bulk_apply.c:4849)
For some reason instead of using a bulk load operation – QR was loading the records one by one. This accounted for the slow performance.
But why was it switching to this one-by-one mode? What caused the main import of bulk insert to fail – but one-by-still works.
Truncating data types
First, I suspected that the column names might be mismatched. I got the source and destination schema out and compared the two.
All the column names aligned correctly.
Turning up the logging we got the following message:
00003568: 2025-02-19T15:24:50 [TARGET_APPLY ]T: Error code 3 is identified as a data error (csv_target.c:1013)
00003568: 2025-02-19T15:24:50 [TARGET_APPLY ]T: Command failed to load data with exit error code 3, Command output: psql:C:/Program Files/Attunity/Replicate/data/tasks/MY_CDC_TASK_NAME/data_files/0/LOAD00000001.csv.sql:1: ERROR: value too long for type character varying(20)
CONTEXT: COPY attrep_changes472AD6934FE46504, line 1, column col12: "2014-08-10 18:33:52.883" [1020417] (csv_target.c:1087)
All the varchar fields between the source and target align correctly.
Then I noticed the column MODIFIED_DATE. On the source it is a datetime; while on the postgres target it is just a date.
My theory was that the bulk copy could not handle the conversion – but in the one-by-one; it could truncate the time component off the date and successfully load.
The downstream team changed the field from a date to a timestamp and I reloaded the data. With this fix the task went blindingly quick; from hours for just a couple of thousands of records to all done within minutes.
Conclusion
I suppose the main conclusion from this exercise is that “Qlik Replicate Knows best.”
Unless you have a very accurate mapping process from the source to the target; let QR create the destination table in a lower environment. Use this table as a source and build on it.
It will save a lot time and heartache later on.